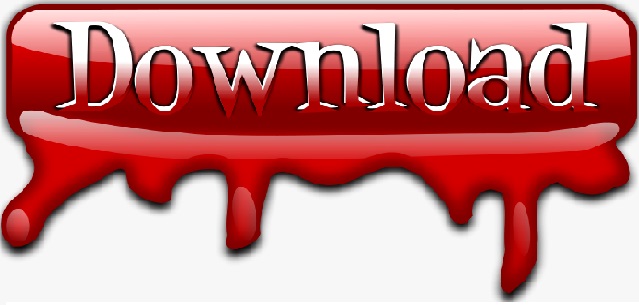
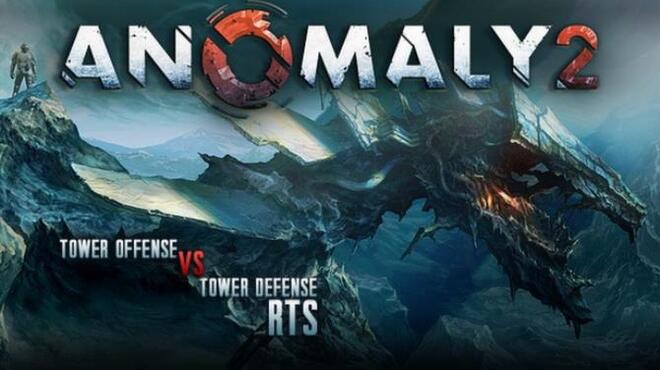
Liu, F.T., Ting, K.M., Zhou, Z.H.: Isolation forest. Lisakov, S.M., Dessart, L., Hillier, D.J., Waldman, R., Livne, E.: Progenitors of low-luminosity Type II-Plateau supernovae. Jones, E., Oliphant, T., Peterson, P., et al.: SciPy: Open source scientific tools for Python (2001). Ishida, E.E.O., et al.: Active Anomaly Detection for Time-Domain Discoveries. Ishida, E.E.O., et al.: Optimizing spectroscopic follow-up strategies for supernova photometric classification with active learning. Hunter, J.D.: Matplotlib: A 2D graphics environment. Henrion, M., Hand, D.J., Gandy, A., Mortlock, D.J.: CASOS: a subspace method for anomaly detection in high dimensional astronomical databases. Guillochon, J., Parrent, J., Kelley, L.Z., Margutti, R.: An open catalog for supernova data. arXiv e-prints arXiv:1809.06477, September 2018ĭas, S., Wong, W.K., Fern, A., Dietterich, T.G., Amran Siddiqui, M.: Incorporating Feedback into Tree-based Anomaly Detection. ĭas, S., Rakibul Islam, M., Kannappan Jayakodi, N., Rao Doppa, J.: Active Anomaly Detection via Ensembles. Ĭooke, J., et al.: Superluminous supernovae at redshifts of 2.05 and 3.90. 93–104 (2000)Ĭontreras, C., et al.: The Carnegie Supernova Project: first photometry data release of low-redshift type ia supernovae. In: Proceedings of the 2000 ACM SIGMOD International Conference on Management of Data, pp. īreunig, M.M., Kriegel, H.P., Ng, R.T., Sander, J.: LOF: identifying density-based local outliers. īentley, J.L.: Multidimensional binary search trees used for associative searching.
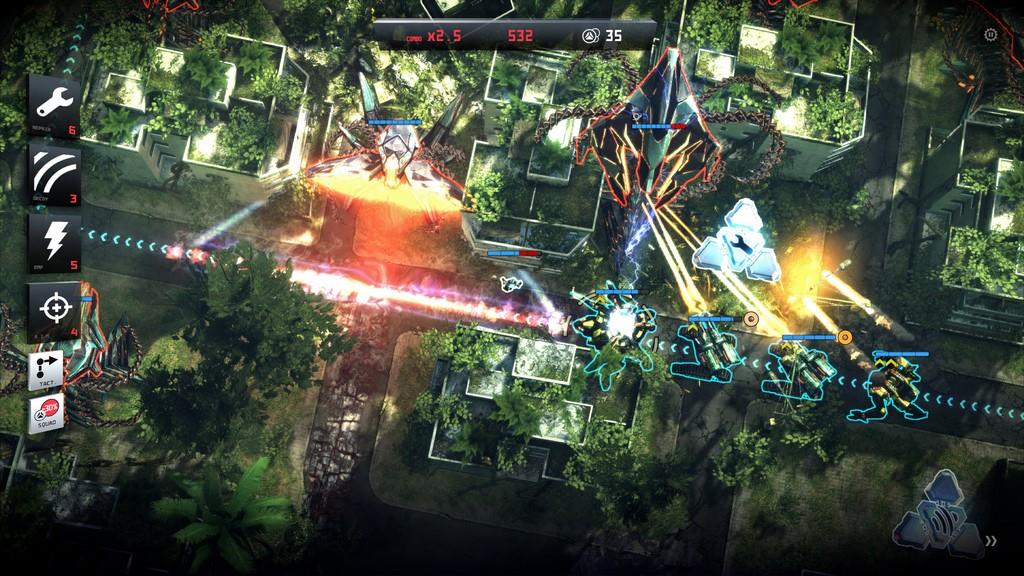
īeck, R., et al.: On the realistic validation of photometric redshifts. īaron, D., Poznanski, D.: The weirdest SDSS galaxies: results from an outlier detection algorithm. The Astronomer’s Telegram 9376, August 2016īall, N.M., Brunner, R.J.: Data mining and machine learning in astronomy. Bakis, V., et al.: Gaia16aye: a flaring object of uncertain nature in Cygnus.
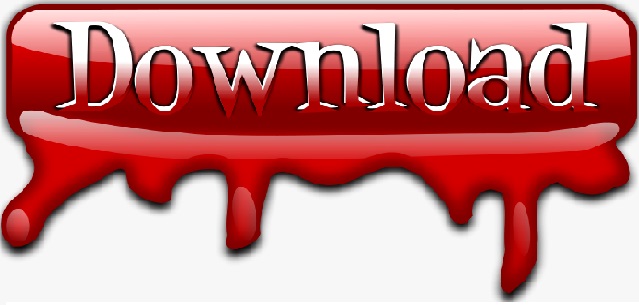